|
Data Science News
|
On Software Demos and Potemkin Villages
|
Wes McKinney
from April 06, 2016
It’s much easier to create impressive demos than it is to create dependable, functionally-comprehensive production software. I discuss my thoughts on this topic.
|
|
DGX-1 Is Nvidia’s Deep Learning System For Newbies
|
The Next Platform
from April 06, 2016
Whenever companies that sell compute components for a living get into the systems business, they usually have a good reason for doing so. There is a delicate balance between addressing a market need quickly and competing with your own channel partners Intel, AMD, and several ARM server chip makers have walked, and Nvidia is toeing that line with its new DGX-1 system for deep learning.
The DGX-1 is not just a server, but a system, and this is a key differentiation that might not be obvious from the way it was presented during the opening keynote at the GPU Technology Conference in San Jose this week.
|
|
Mapping the Brain to Build Better Machines | Quanta Magazine
|
Quanta Magazine, Emily Singer
from April 06, 2016
Visual identification is one of many arenas where humans beat computers. We’re also better at finding relevant information in a flood of data; at solving unstructured problems; and at learning without supervision, as a baby learns about gravity when she plays with blocks. “Humans are much, much better generalists,” said Tai Sing Lee, a computer scientist and neuroscientist at Carnegie Mellon University in Pittsburgh. “We are still more flexible in thinking and can anticipate, imagine and create future events.”
An ambitious new program, funded by the federal government’s intelligence arm, aims to bring artificial intelligence more in line with our own mental powers. Three teams composed of neuroscientists and computer scientists will attempt to figure out how the brain performs these feats of visual identification, then make machines that do the same.
|
|
This Startup Is Predicting the Future by Decoding the Past
|
Bloomberg Business
from April 06, 2016
When James Shinn was working for the CIA as a senior East Asia expert more than a decade ago, he longed for the tools of a weatherman. He wanted to be able to predict that the chance of North Korea test-firing a missile within a month was, say, 60 percent. It remained a fantasy, he says, until now.
Shinn and his 14-person team at Predata have developed software that numerically describes political volatility and risk. It vacuums up vast quantities of data from online conversations and comments, compares them with past patterns, and spits out a probability. (A version of Predata’s service is accessible on the Bloomberg Professional service.) Shinn likens his product to sabermetrics, the statistics-driven baseball strategy popularized in Michael Lewis’s Moneyball. “By carefully gathering lots and lots of statistics on their past performance from all corners of the Internet, we are predicting how a large number of players on a team will bat or pitch in the future,” Shinn says, by way of analogy.
|
|
Fast Forward Labs: Where Do You Put Your Data Scientists?
|
Fast Forward Labs Blog, Daniel Tunkelang
from April 06, 2016
… Difficult as it is to hire data scientists, it’s only the first step. Once you find great data scientists, where do you put them in your organization to set them up for success?
As companies increasingly rely on data as a core asset, a data science team can deliver enormous value by enabling companies to build better products and make better decisions using that data. So it’s not surprising that many executives are convinced they need data scientists yesterday.
But those same executives often don’t know how to hire data scientists, where to put them, or what to do with them.
|
|
Self-regulatory information sharing in participatory social sensing
|
EPJ Data Science journal; Evangelos Pournaras et al.
from April 01, 2016
Participation in social sensing applications is challenged by privacy threats. Large-scale access to citizens’ data allow surveillance and discriminatory actions that may result in segregation phenomena in society. On the contrary are the benefits of accurate computing analytics required for more informed decision-making, more effective policies and regulation of techno-socio-economic systems supported by ‘Internet-of Things’ technologies. In contrast to earlier work that either focuses on privacy protection or Big Data analytics, this paper proposes a self-regulatory information sharing system that bridges this gap. This is achieved by modeling information sharing as a supply-demand system run by computational markets. On the supply side lie the citizens that make incentivized but self-determined decisions about the level of information they share. On the demand side stand data aggregators that provide rewards to citizens to receive the required data for accurate analytics. The system is empirically evaluated with two real-world datasets from two application domains: (i) Smart Grids and (ii) mobile phone sensing. Experimental results quantify trade-offs between privacy-preservation, accuracy of analytics and costs from the provided rewards under different experimental settings. Findings show a higher privacy-preservation that depends on the number of participating citizens and the type of data summarized.
|
|
[1603.09326] Estimating Treatment Effects using Multiple Surrogates: The Role of the Surrogate Score and the Surrogate Index
|
arXiv, Statistics > Methodology; Susan Athey et al.
from March 30, 2016
Estimating the long-term effects of treatments is of interest in many fields. A common challenge in estimating such treatment effects is that long-term outcomes are unobserved in the time frame needed to make policy decisions. One approach to overcome this missing data problem is to analyze treatments effects on an intermediate outcome, often called a statistical surrogate, if it satisfies the condition that treatment and outcome are independent conditional on the statistical surrogate. The validity of the surrogacy condition is often controversial. Here we exploit that fact that in modern datasets, researchers often observe a large number, possibly hundreds or thousands, of intermediate outcomes, thought to lie on or close to the causal chain between the treatment and the long-term outcome of interest. Even if none of the individual proxies satisfies the statistical surrogacy criterion by itself, using multiple proxies can be useful in causal inference. We focus primarily on a setting with two samples, an experimental sample containing data about the treatment indicator and the surrogates and an observational sample containing information about the surrogates and the primary outcome.
|
|
Moderating Harassment in Twitter with Blockbots
|
Berkeley Institute for Data Science, R. Stuart Geiger
from April 06, 2016
I’ve been working on a research project about counter-harassment projects in Twitter, where I’ve been focusing on blockbots (or bot-based collective blocklists) in Twitter. Blockbots are a different way of responding to online harassment, representing a more decentralized alternative to the standard practice of moderation—typically, a site’s staff has to go through their own process to definitively decide what accounts should be suspended from the entire site. I’m excited to announce that my first paper on this topic will soon be published in Information, Communication, and Society.
|
|
How economists rode maths to become our era’s astrologers
|
Aeon Essays, Alan Jay Levinovitz
from April 04, 2016
… it’s a bull market for academic economists. According to a 2015 sociological study in the Journal of Economic Perspectives, the median salary of economics teachers in 2012 increased to $103,000 – nearly $30,000 more than sociologists. For the top 10 per cent of economists, that figure jumps to $160,000, higher than the next most lucrative academic discipline – engineering. … Unlike engineers and chemists, economists cannot point to concrete objects – cell phones, plastic – to justify the high valuation of their discipline.
|
|
NVIDIA CEO on Emergence of a New Computing Model
|
The Next Platform
from April 05, 2016
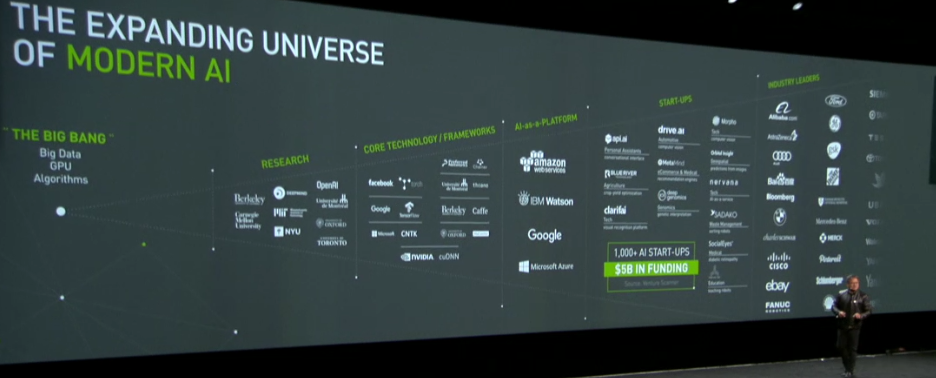
It is hard to find a more hyperbolic keynote title than, “A New Computing Model” but given the recent explosion in capabilities in both hardware and algorithms that have pushed deep learning to the fore, Nvidia’s CEO keynote at this morning’s GPU Technology Conference kickoff appears to be right on target.
|
|
OpenAI, Hyperscalers See GPU Accelerated Future for Deep Learning
|
The Next Platform
from April 06, 2016
As a former research scientist at Google, Ian Goodfellow has had a direct hand in some of the more complex, promising frameworks set to power the future of deep learning in coming years.
He spent his first years at the search giant chipping away at TensorFlow, creating new capabilities, including the creation of a new element to the deep learning stack, called generative adversarial networks. And as part of the Google Brain team, he furthered this work and continued to optimize machine learning algorithms used by Google and now, the wider world. Goodfellow has since moved on to the non-profit OpenAI company, where he is further refining what might be possible with generative adversarial networks.
The mission of OpenAI is to develop open source tools to further many of the application areas that were showcased this week at the the GPU Technology Conference this week in San Jose, where the emphasis was placed squarely on the future of deep learning.
|
|
Events
|
2016 Atlantic Causal Inference Conference
The Atlantic Causal Inference Conference is a gathering of statisticians, biostatisticians, epidemiologists, economists, social science and policy researchers to discuss methodologic issues with drawing causal inferences from experimental and non-experimental data. The inaugural meeting was held in 2005 with a small group of researchers at Columbia University and has since grown into an annual event with over 100 attendees.
New York, NY. Thursday-Friday, May 26-27, at New York University, Kimmel Center
|
|
Deadlines
|
NOT-OD-16-043: NIH and FDA Request for Public Comment on Draft Clinical Trial Protocol Template for Phase 2 and 3 IND/IDE Studies
|
deadline: subsection?
|
The National Institutes of Health (NIH) and Food and Drug Administration (FDA) are developing a template with instructional and sample text for NIH funded investigators to use in writing protocols for phase 2 or 3 clinical trials that require Investigational New Drug application (IND) or Investigational Device Exemption (IDE) applications. The agencies’ goal is to encourage and make it easier for investigators to prepare protocols that are consistently organized and contain all the information necessary for the clinical trial to be properly reviewed. The draft template follows the International Conference on Harmonisation (ICH) E6 Good Clinical Practice.
NIH and FDA are seeking public comment on the draft template available at: http://osp.od.nih.gov/office-clinical-research-and-bioethics-policy/clinical-research-policy/clinical-trials.
Deadline to respond is Sunday, April 17.
|
|
HILDA 2016: Workshop on Human-In-the-Loop Data Analytics
|
deadline: subsection?
|
HILDA is a new workshop that will allow researchers and practitioners to exchange ideas and results relating to how data management can be done with awareness of the people who form part of the processes. A sample of topics that is are in the spirit of this workshop includes, but is not limited to: novel query interfaces, interactive query refinement, data exploration and analysis, data visualization, human-assisted data integration and cleaning, perception-aware data processing, database systems designed for highly interactive use cases, empirical studies of database use, and crowd-powered data infrastructure. (Co-located with SIGMOD 2016 in San Francisco, CA.)
Deadline for submissions is Monday, April 18.
|
|
Round 4 | Digging Into Data
|
deadline: subsection?
|
The T-AP Digging into Data Challenge will launch in March 2016. The Challenge will support research projects that explore and apply new “big data” sources and methodologies to address questions in the social sciences and humanities.
The funding opportunity is open to projects that address any research question in humanities and/or social sciences disciplines by using new, large-scale, digital data analysis techniques. All projects must show how these techniques can lead to new theoretical insights. Proposed projects can use any data source.
Deadline to apply is Wednesday, June 29.
|
|
Tools & Resources
|
?hat | Data Normalization in Python
|
?hat blog, Greg Lamp
from April 06, 2016
Well it’s that time of the year again in the United States. The 162 game marathon MLB season is officially underway. In honor of the opening of another season of America’s Pasttime I was working on a post that uses data from the MLB. What I realized was that as I was writing the post, I found that I kept struggling with inconsistent data across different seasons. It was really annoying and finally it hit me: This is what I should be writing about! Why not just dedicate an entire post to normalizing data!
So that’s what I’ve done. In this post we’ll be digging into some MLB payroll data. In particular I’m going to show you how you can use normalization techniques to compare seemlingly incomparable data! Sounds like magic? Well it’s actually really simple, but I think these little Python scripts will really help you out 🙂
|
|
Careers
|
|
Doing Data Science Right — Your Most Common Questions Answered
First Round Review, Jeremy Stanley and Daniel Tunkelang
|
|
10 Signs Of A Bad Data Scientist
KDnuggets, Seamus Breslin
|
|
Data Science Job Requirements and Training Advice from Wayfair
BostInno
|
|